Jonas Hübotter
Doctoral Researcher at ETH Zurich. I work on Active Fine-Tuning and Local Learning.
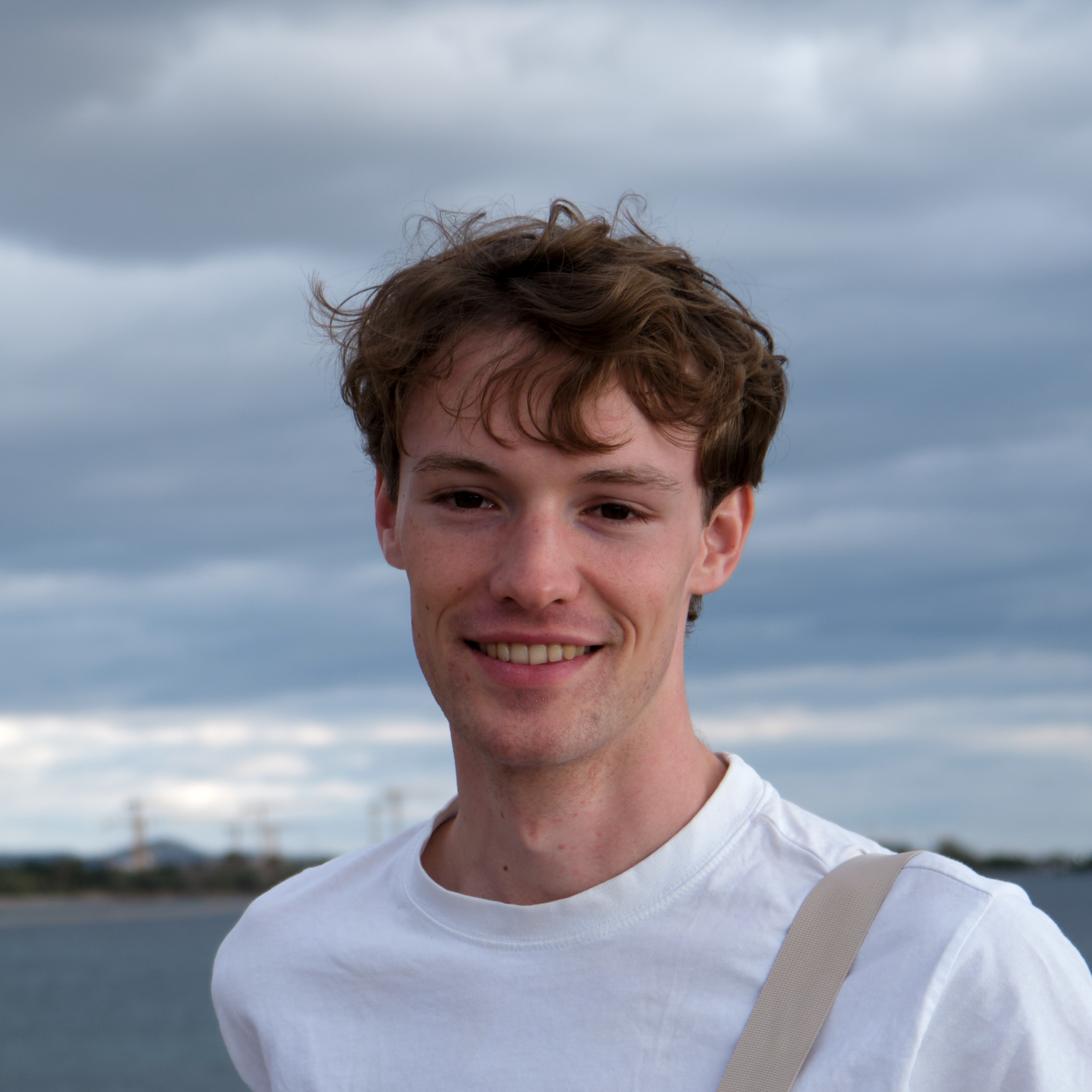
I am a doctoral researcher in the Learning and Adaptive Systems Group at ETH Zurich working with Andreas Krause. Prior to this, I obtained a Master’s degree in Theoretical Computer Science and Machine Learning from ETH Zurich and a Bachelor’s degree in Computer Science and Mathematics from the Technical University of Munich. As an intern at Citadel Securities, I have previously worked with Guillaume Basse and Sören Künzel on time-series prediction. I am a recipient of the ETH Medal.
My research aims to improve the performance of foundation models by utilizing tools from active learning for few-shot learning, active inference, and adaptive computation. Beyond this, I have broad interests including (approximate) probabilistic inference, optimization, and online learning.
Always feel free to reach out to me with things you find exciting.
Contacts: jhuebotter@ethz.ch Google Scholar GitHub Linkedin
Announcements
Oct, 2024 | NeurIPS 2024: Our work Transductive Active Learning: Theory and Applications was accepted! We will also present our work on efficiently learning at test-time with LLMs with an oral presentation at the Fine-Tuning in Modern ML workshop. |
---|---|
Jun, 2024 | ICML 2024: Our work on Transductive Active Learning with Application to Safe Bayesian Optimization was accepted as an oral presentation (top 5%) at the Aligning RL Experimentalists and Theorists workshop. |
Mar, 2024 | ICLR 2024: Our work on Active Few-Shot Fine-Tuning was accepted at the Bridging the Gap Between Practice and Theory in Deep Learning workshop! |
Feb, 2024 | I received the ETH Medal for my Master’s thesis on transductive active learning 🎉! Big thanks to my incredible collaborators Bhavya Sukhija, Lenart Treven, Yarden As, and Andreas Krause. |
Selected Publications
Talks
- Efficiently Learning at Test-Time: Active Fine-Tuning of LLMs
Contributed Talk, NeurIPS Workshop on Fine-Tuning in Modern Machine Learning, Vancouver, 14 Dec 2024. - Transductive Active Learning for Fine-Tuning Large (Language) Models — slides
Invited Talk, Machine Learning and Modelling Seminar, Charles University Prague, 21 Nov 2024. - Efficiently Learning at Test-Time with LLMs — recording, slides
Invited Talk, Zurich AI Meetup, Zurich, 3 Dec 2024.
Invited Talk, Tufa Labs AI Meetup, Zurich, 29 Oct 2024. - Transductive Active Learning with Application to Safe Bayesian Optimization — recording, slides
Contributed Talk, ICML Workshop on Aligning Reinforcement Learning Experimentalists and Theorists, Vienna, 26 Jul 2024. - Active Fine-Tuning of Large Neural Networks — slides
Contributed Talk, Machine Learning Seminar, ETH Zurich, 18 Apr 2024.
Supervision
I have had the privilege of advising several BSc and MSc students during their theses and semester projects.
- Nicolas Menet: Efficiently Estimating Gaussian Probability of Maximality (with Parnian Kassraie)
- Sascha Bongni: Active Fine-Tuning of Large Language Models
- Pablo Lahmann: Safe Control as Inference (with Yarden As)
- Anh Duc Nguyen: Safe Bayesian Optimization without Regret
Fun Projects
Efficiently fine-tune large neural networks by intelligent active data selection.
Algorithms for online convex optimization with an associated cost for movement in the decision space. Useful for resource allocation, contextual sequence prediction, portfolio management, and object tracking.
Solutions to a wide variety of competitive programming-type questions.
A serverless web app to organize and stream media from anywhere.
Website of a landscape architecture firm.